Topology Inspired Methods for the Design and Analysis of Transcriptomic Time-Series Data in Circadian Biology Research
Public Deposited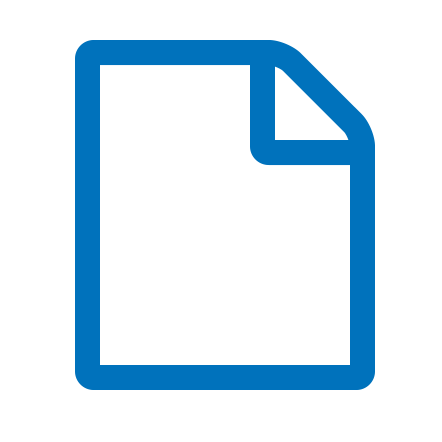
Circadian rhythms — physiological, behavioral, and metabolic oscillations with an approximate 24-h period — are controlled by an evolutionarily conserved set of core clock genes operating at the transcriptional and protein level. Entrainable by Zeitgebers (external environmental stimuli such as light, temperature, and food) that modulate time-of-day specific functions, the circadian rhythm is a self-sustained molecular oscillator that orchestrates a multitude of cellular processes, including nearly half of genes across all tissues. Although numerous epidemiological studies have established significant links between circadian rhythms and human health, the underlying biological mechanisms coupling these phenomena remain poorly understood. Facilitated by the development of high-throughput assays, researchers can now interrogate circadian regulation at the molecular level by analyzing transcriptomic time-series data to identify genes under circadian control. Typically, such experiments comprise RNA-seq on samples collected every 2-4h across a time span of 24-48h with 1-3 replicates. Computational methods to quantify and compare rhythmic patterns in such data must overcome the challenges of sparse temporal sampling, noisy measurements, and dynamics that may not be strictly sinusoidal. While a variety of cycling detection methods have been proposed, results from our user-friendly web application — as well as other bench-marking studies — indicate that no method is universally optimal. Additionally, there remains a need for differential cycling analysis methods that can identify complex changes in rhythmicity without making assumptions of simple sinusoidal dynamics. To address these challenges, we introduce a suite of methods that exploit results from dynamical systems theory and topological data analysis for the analysis of circadian transcriptomic data: TimeCycle (for the detection of cycling genes) and TimeChange — powered by an R implementation of the Fasano-Franschechini test — (for the quantification of differential rhythmicity). Crucially, both methods are non-parametric and model-free, and can be applied without any assumptions about the underlying dynamics. This thesis outlines the methods and demonstrate their results in synthetic and real applications.
- Last modified
- 01/20/2022
- Creator
- DOI
- Publisher
- Keyword
- Date created
- Resource type
- License
- Total Size
- 0 Bytes